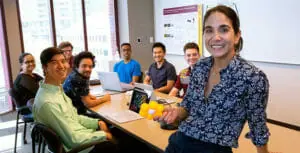
Download Photo
Some things seem to happen without direction. Fish form schools to deter predators and ants form rafts to survive floods. These emergent group behaviors have long been the focus of research in biological science, but they are inspiring new work in computing and robotics.
Members of the Biodesign Center Biocomputing, Security and Engineering Andréa Richa, a professor of computer science in the Ira A. Fulton Schools of Engineering, and Joshua Daymude, a postdoctoral researcher, are exploring ways that algorithms can help explain how local interactions induce large-scale phenomena without top-down direction.
“There is plenty of great robotics work in progress related to self-organizing groups. Think of swarms of drones or autonomous vehicles,” Daymude said. “But what happens when you go down to the micro- or nanoscale and take away the processing capability that comes from microprocessors and sensors? Can we approximate the activity of groups of devices that have no coordinating intelligence?”
The answer appears to be yes. Together with professors of computer science Dana Randall at Georgia Tech and Sarah Cannon at Claremont McKenna College, Richa and Daymude devised an algorithm that controls how collections of abstract particles move on a lattice. This computational model accurately predicted the behavior of 30 “dumb” robots in a physical experiment. The successful result opens opportunities for innovation in fields ranging from manufacturing to medicine.
“Imagine someone suffering from internal bleeding, and surgery is not a timely option,” Richa said. “This line of work could lead to the development of tiny particles known as colloidal state machines that the patient can swallow, and they’ll swarm through the person’s system to rapidly fix the problem.”
The exciting new findings have just been published in the journal Science Advances.
Daniel Goldman, a professor of physics at Georgia Tech, and first co-author students Shengkai Li and Bahnisikha Dutta created the group of basic robots to serve as the physical proof for the computational model that Richa and Daymude developed at ASU. Each one was a 4-centimeter (or 1.5-inch) plastic puck embedded with loose magnetic beads and mounted on tiny bristle brush feet. Vibration from a small electric motor enabled noisy, circular motion across a 1.2-meter by 0.75-meter (or 4-foot by 2.5-foot) test platform.
Across multiple experiments, the strength of the embedded magnets was gradually increased to transition the particles (in the simulation) and the robots (in the physical proof) from a scattered state to a congregated one. That magnetism was the sole input or “bias parameter.”
“It mimics how intently theoretical particles, or physical robots, seek to be near others. And you might expect there would have been a steady increase in aggregate grouping as we increased that magnetism,” Daymude said. “But that isn’t what we saw in our computational model nor in the experimental robot platform.”
Instead, nothing changed as the bias increased. The particles and the robots stayed largely spread out in their movement — until a particular threshold was achieved, and then aggregates formed rapidly. Collective behavior emerged like fish forming a school.
“We saw a sharp phase change from dispersion to aggregation, just as our theory predicted,” Richa said. “We usually think about phase changes in physics, but they’re in fact more universal. Our inspiration comes from biological and physical systems, like groups of animals acting in unison. What they do collectively is much more powerful than what any of them could do alone. So, we are seeking a better algorithmic understanding of collective behavior since it represents the potential to manipulate local actions and achieve desired outcomes without centralized direction.”
The paper represents pioneering ground for computer science, physics and robotics. It is a very early effort in the translation of computational abstractions as a mechanism for programming through physics or embedded physical characteristics rather than through digital code.
It also is part of a larger emergent computation research project that began in 2019 with ASU, Georgia Tech, Massachusetts Institute of Technology and Northwestern University through funding from a U.S. Department of Defense program called the Multidisciplinary University Research Initiative.
“And while this particular result was directed toward a behavior we wanted to achieve, you could flip the orientation,” Daymude said. “This framework can generate local rules that act as scientific tools to analyze the behavior of complex systems that we don’t really understand right now. So, these techniques can support a broad range of technical solutions, but they can also advance what we know about social and biological systems.”